Inferring meaning using synthetic language AI
By Dr Andrew D Back, TAS AQ Fellow
How can AI understand what I really mean?
Future AI will need more human-like capabilities such as being able to infer intent and meaning by understanding behaviors and messages in the real world. This will include being able to detect when someone is saying one thing but meaning another, when spoken messages are saying one thing but our observations make us question what is really happening. We need to first come to understand what we mean by meaning.
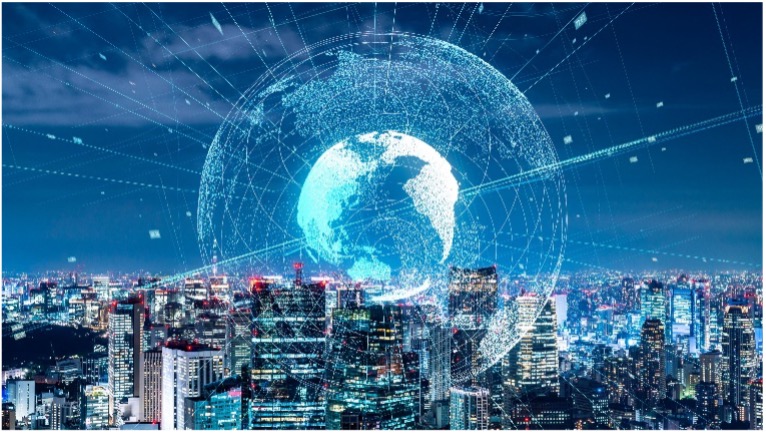
Fig. 1 In a world where communications is so prevalent, how do we understand the true meaning and importance of what is being said?
Meaning is often thought of in absolute terms, yet from human experience, we know that there are many levels of meaning. For example, a person says “I am going to eat an orange” or “yes I agree”, the meaning is clear at the surface level, but we can also infer additional meaning with background knowledge. In the first case, the person might be inferring something completely different than the mere act of eating a piece of fruit, perhaps they are recovering from serious illness, so this is conveying that they are recovering their health and independence. In the second case, how do we really know whether there is agreement?
In general, the aims of discovering meaning in AI has often been in the context of natural language processing. For example, Alexa hears a command and then acts on the precise meaning of the words “turn on the light”. But there is much more to meaning that simple translation of commands.
A problem for AI is being able to move beyond simple interpretation of inputs to assessing inputs within context. A picture might look like it is of a penguin, or the person might have said one thing, but is that really the case? How can AI assess multiple layers of meaning? A direct translation might seem very simple, but can readily become caught up in the semantics of human language. We know that meaning is dependent on issues such as context, attention, background knowledge and expectations.
Many would be familiar with the children’s game where you are shown a number of different items and then later asked to remember what you have seen. It’s difficult to recall beyond a small number. As humans we can read a situation very well in many cases but then miss it completely when there is too much data to comprehend.
In modern life and particularly in areas of security and defence where there can be a wide range of data with complex socio-political systems, with too much for humans to quickly and fully comprehend [1], it is imperative to make assessments or risk significant consequences [2].
So how can AI be equipped to understand true meaning in complex social, geo-political or socio-political situations [3]? We can comprehend what may be important without necessarily knowing the full picture, but how do we translate these ideas to AI? How do we build AI systems that can infer meaning? Is it possible to define meaning in a mathematical framework so that it can be implemented reliably in an AI system?
The problem that hasn’t yet been solved
Despite incredible successes, a problem with AI systems since 1970s and even to the present is that they are brittle, which means that they have difficulty in adapting to new scenarios and displaying more intuitive, general intelligence properties [4,5]. Another significant problem is that they are opaque, which means that when they do something, we often do not know why or how they arrived at their decision. In addition, current AI systems require enormous amounts of data and computation, yet we envisage that there is a need for AI to make robust decisions quickly and with scant data in much the same way that humans are often called to do.
The reason for these problems in AI is essentially two-fold: present AI systems tend to focus on the infinite richness of human expression which leads to increasingly more complex networks and vast data requirements. As this is done to achieve results, it becomes increasingly difficult to understand what the AI system has learned [6].
Regular machine learning can have difficulties when it doesn’t have a sense of priorities, wider contextual importance of some events over others, and of overall connectedness where the pieces make up a “story” of thematic information. In essence, machine learning systems have become very good at recognizing images, but without knowing what those images mean or if they can be trusted.
These problems are compounded when trying to understand the relationships between events, their distance, connectedness and behaviors, yet this can be difficult and completely overlooked unless it is precisely trained for. But how can we train to look out for something we haven’t seen? Hence the problem for AI is to understand that meaning is inextricably linked to inter-related behaviors, not just learned features.
A new AI paradigm
To address this, we have proposed a new concept in AI called synthetic language, which has the potential to overcome these limitations. In this new approach, almost every dynamic system of behavioral interaction can be viewed as having a form of language [9-11]. We are not referring necessarily to human interaction, or even human language through natural language processing, but a far broader understanding of language, including novel synthesized languages which describe the behaviors of particular systems. Such languages might define the characteristics of artificial or natural systems using a linguistic framework in ways which have not been considered before.
Synthetic language is based on the idea that there can exist ‘conversations’ or dialogs where the alphabet size may be very small, perhaps only 5-10 symbols and yet still provide a rich framework in terms of vocabulary and topics where we can identify situations very rapidly, without the long training times typically associated with machine learning.
We suggest that in the context of synthetic language, a powerful construct is the idea of relative meaning, not necessarily at the human language level. For instance, we would like to understand the meaning of behavioral interactions at a socio-political level, the synthetic language approach will effectively enable us to learn about the changes taking place in a relative sense which is meaningful in itself. Even knowing when something unusual has occurred is a potentially important task.
Autonomously recognizing when something ‘looks different’ without training on specific examples when humans cannot comprehend quickly enough is likely to be particularly advantageous in defence applications [7]. A key advantage of the synthetic language AI approach is that it has the potential to be used autonomously to uncover hidden meaning in the form of tell-tale signs and true intent that humans might otherwise miss [8].
Foreign relations: how can synthetic language AI help?
As an example of the types of problems we are interested in, suppose we would like to understand the true intent and meaning of states or state actors. In addition to diplomatic statements, there is a potential over-abundance of data coming from a wide range of sources such as military data, observational data, news sources, social media, operational data, intelligence reports, climate information and historical data [2].
We could apply synthetic language AI to consider behavioral interactions at a socio-political level, which form a meta-dialog or story. The hidden messaging elements of diplomatic statements, subtle behaviors of military forces such as relatively small changes of aircraft flight paths, and even hostile acts such as cyber-attacks form a synthetic language which can be compared against each other to determine areas of significance.
By comparing visible and invisible behavioral dialogs across multiple sources, synthetic language gives the possibility of assessing whether there are mixed messages, such as rhetorical bluster versus a potential hidden intent and in so doing, make assessments about potential threats (Fig. 2). If there is a consistency or even leading indicators of subtle events detected in say, operational behavior, this might add weight to diplomatic statements.
On the other hand, if diplomatic statements appear similar to their usual behavioral characteristics then there may be less cause for alarm, despite the particular rhetoric. Clearly this approach can be extended to a wider range of sources, with the aim of detecting anomalies, where there is a departure from the usual behaviors, whether more or less calm for example.
Adopting this new synthetic language approach provides the scope for understanding hidden messages and gaining meaningful insights into behaviors of complex systems without necessarily knowing what we ought to look for. Detection of socio-political anomalies in this way can be further processed at next level of AI and escalated as required to human analyses in an explainable way for further consideration.
![]() |
![]() |
Fig. 2 Synthetic language provides the potential capability of detecting tell-tale signatures of unusual behaviors. For example, comparing the hidden synthetic language of official diplomatic statements against operational synthetic language and hostile synthetic language dialogs, we can potentially infer the deeper intent, such as rhetoric or otherwise. The red curve represents some hidden synthetic language which might appear in operational events such as flight paths, while the blue curve represents some visible synthetic language which might add weight to diplomatic statements. |
References
- Gregory C. Allen, Understanding China’s AI Strategy: Clues to Chinese Strategic Thinking on Artificial Intelligence and National Security, Center for a New American Security, pp. 3-4, 2019.
- Thomas K Adams, ‘Future Warfare and the Decline of Human Decision making,’ The US Army War College Quarterly: Parameters 41, No. 4, p. 2., 2011.
- Malcolm Davis, ‘The Role of Autonomous Systems in Australia’s Defence,’ After Covid-19: Australia and the world rebuild (Volume 1), pp. 106-09, 2020.
- James Lighthill, “Artificial Intelligence: A General Survey”, in J. Lighthill, N.S. Sutherland, R.M Needham, H.C. Longuet-Higgins and D. Michie (editors), Artificial Intelligence: a Paper Symposium, Science Research Council, London, 1973.
- Douglas Heaven, “Why deep-learning AIs are so easy to fool”, Nature, pp. 163-166, 574 (7777), Oct, 2019.
- Greg Allen, Understanding AI technology, Joint Artificial Intelligence Center (JAIC) The Pentagon United States, 4, 2020.
- Glenn Moy et al., Recent Advances in Artificial Intelligence and their Impact on Defence, 25 (Canberra 2020).
- Malcolm Gladwell, Talking to Strangers: What We Should Know about the People We Don’t Know, Little, Brown & Co, 2019.
- A. D. Back and J. Wiles, “Entropy estimation using a linguistic Zipf-Mandelbrot-Li model for natural sequences”, Entropy, Vol. 23, No. 9, 2021.
- A. D. Back, D. Angus and J. Wiles, “Transitive entropy — a rank ordered approach for natural sequences”, IEEE Journal of Selected Topics in Signal Processing, Vol. 14, No. 2, pp. 312-321, 2020.
- A. D. Back, D. Angus and J. Wiles, “Determining the number of samples required to estimate entropy in natural sequences”, IEEE Trans. on Information Theory”, Vol. 65, No. 7, pp. 4345-4352, July 2019.
- A.D. Back and J. Wiles, “An Information Theoretic Approach to Symbolic Learning in Synthetic Languages”, Entropy 2022, 24, 2, 259.